Q&A: Donald Thompson, Distinguished Engineer, LinkedIn

(Editor's Note: We conduct regular Q&As with technology leaders as part of our Cloud Tracker Pro [CTP] service. We are offering this Q&A for a limited time, before it becomes part of the CTP subscription.)
Donald Thompson is currently a Distinguished Engineer at LinkedIn, primarily overseeing the company's generative AI strategy, architecture, and technology. He has more than 35 years of hands-on experience as a technical architect and CTO, with an extensive background in designing and delivering innovative software and services on a large scale.
In 2013, Donald co-founded Maana, which pioneered computational knowledge graphs and visual no-code/low-code authoring environments to address complex AI-based digital transformation challenges in Fortune 50 companies.
During his 15 years at Microsoft, Donald started the Knowledge and Reasoning group within Microsoft's Bing division, where he innovated "Satori," an Internet-scale knowledge graph constructed automatically from the web crawl. He co-founded a semantic computing incubation funded directly by Bill Gates, portions of which shipped as the SQL Server Semantic Engine. Additionally, he created Microsoft's first Internet display ad delivery system and led numerous AI/ML initiatives in Microsoft Research across embedded systems, robotics, wearable computing, and privacy-preserving personal data services.
Futuriom conducted this interview via email. It was delivered early in January 2025.
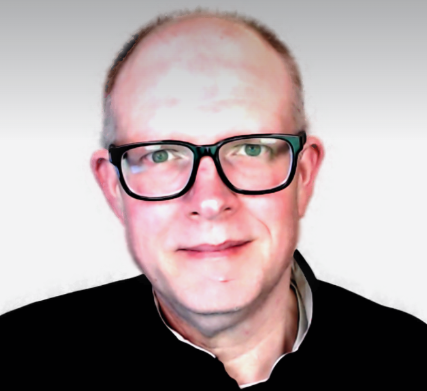
Donald Thompson.
To access the rest of this article, you need a Futuriom CLOUD TRACKER PRO subscription — see below.
Access CLOUD TRACKER PRO
|
CLOUD TRACKER PRO Subscribers — Sign In |
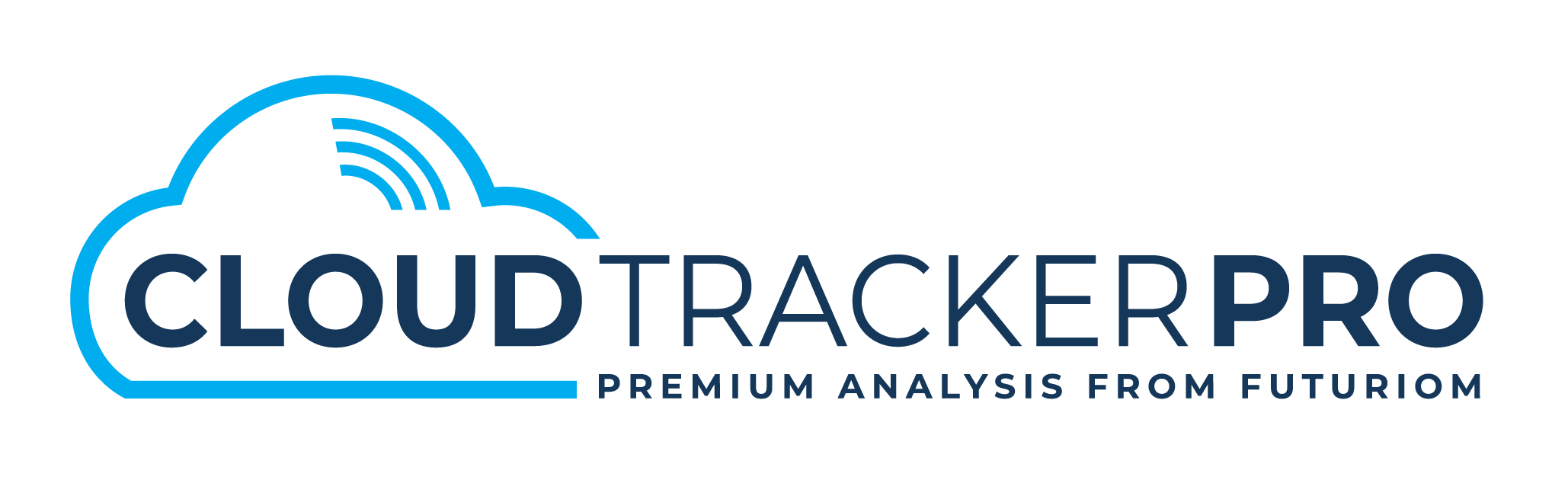